Seeking Alpha in Private Company Data & Analytics
TL;DR - Are Expert Networks the future of Company Data & Analytics?
My last two posts have mainly focused on AltData - data that enables traders to make Alpha-generating decisions.
While writing my last newsletter, I started to think about data on non-public (AKA private!) companies, and how you might distinguish between providers of Alpha and non-Alpha data in this space. Indeed, one of my readers, the Head of High Yield Trading at a global investment bank (you know who you are), sent me a message musing along the same lines.
Sounds like an appropriate subject for my next post, I thought.
So here we go!
(Apologies in advance for focusing in the main here on how Company Data & Analytics is of use to a segment of financial services companies: the investment community and its attendant advisors. It is, of course, valuable to other businesses too, but I’ll leave that for another post.)
Statista estimated that there were 333 million companies globally in 2021, and that number is growing all the time.
Out of that 333 million total, only c.58,200 were public companies. That’s 0.000174% of the total. Even if you round up, that’s one company in every c.5,000!
So the private company space is absolutely vast.
The Company Data & Analytics sector, providing data on all companies (public and private), has been my bread and butter for the past 16 years.
I have thought about this sector a lot over the years for a number of reasons:
From 2018 to 2023 I built a 45-person global team in large part focused on creating an enormous dataset of company data at Houlihan Lokey, the largest Corporate Finance advisor by volume globally;
Corporate Finance is interested in all companies, not just public companies;
Private Equity has become the most influential player in M&A, and non-listed businesses make up the vast majority of PE investments, bolt-ons and potential acquisition targets;
The number of public companies has been in continuous decline in recent years. PE firms are more inclined to sell to one another (or themselves (FT: subscription required)) than to float their investments;
At Quayle Munro (where I worked from 2008 until 2018 until it was acquired by Houlihan Lokey) we spent a lot of time serving the Company Data market as sell-side M&A advisors;
Back in 2012 I started a Company Data business (shuttered in 2015), so I know a few things about the pitfalls of the sector!
In my view you’re better off thinking about any market with a bottom-up approach (build the wall brick by brick, company by company) than top-down (look at the whole wall and try to guesstimate the size of the market). To do this you need information on each company.
I’m an information magpie…
The Company Data & Analytics market evolved out of the directory businesses of the previous century. And the taxonomy used to categorise “industries” fitted with that paper-based system. SIC and NAIC codes are products of an analogue way of thinking.
I first started working in Corporate Finance 16 years ago, coming from a background in technology (Web Analytics, an MA in building online information systems). When I began to use the Company Data & Analytics products on the market, I was shocked by the low quality of solutions provided.
Little effort had been made to answer the key issues:
Discoverability - how do you surface the most relevant companies out of a dataset?
Accuracy - how do you ensure the data is not full of junk?
Workflow - how do you fit the data into the workflow of the person who needs it?
Most Company Data & Analytics providers at this time simply combined company registry data (e.g. Companies House in the UK) with SIC/NAIC codes for discoverability, plus perhaps some small amount of proprietary data, usually licensed in from a third party like D&B Hoovers (the top choice for alternative private company data back in 2008). Revenue data was extremely poor to say the least - little attempt had been made to estimate the size of companies accurately.
Fast forward to 2023 and progress has been made. In large part, I would say, this is due to the integration of what we might deem Alternative Data into Company Data to make it richer and more useful.
SIC and NAIC codes are now largely ignored as useful tools for discovery in favour of (increasingly AI-driven) proprietary taxonomies;
Company websites and other directories have provided Company Data & Analytics providers with resources from which they can scrape descriptions, product information and contact details;
Company Data & Analytics providers have woken up to the need for financial information to be as accurate as possible. The biggest change here is the use of employee data from LinkedIn to estimate company revenues.
Although LinkedIn already existed back in 2008, it had not reached its current scale. LinkedIn now provides a master database of company information for much of the western world (certainly English-speaking world). Data is kept (fairly) reliably up to date by the companies and individuals in LinkedIn themselves.
But LinkedIn, with their focus on contacts rather than companies, have not yet properly productised their data as Company Data & Analytics. Individuals can use Sales Navigator to access the data but it’s painful and time-consuming to integrate this information with data from a Company Data & Analytics provider. (It’s hard to tell whether LinkedIn are protecting their user base or just missing the point).
This has opened up a gap in the market for companies like SourceScrub, who derive a lot of their value from mining LinkedIn for sizing information and reselling it to grateful researchers.
But is this really Alpha? Does it really move the needle in terms of understanding companies?
I guess that in the terms we defined last time, where Alpha data is data that others don’t have that means you can make better decisions than them, then yes it is. Or at least it has been. But if every corporate finance advisor, private equity firm and VC now has Pitchbook and SourceScrub, then are these data sources now just table stakes?
So, what does Alpha now look like in the Company Data space?
I would posit that it looks like companies that are attempting to add value in the same three areas I outlined above: discoverability, accuracy and workflow.
I’m spending a lot of my time at the moment out in the real world talking to investors, advisors and business owners, the majority of whom have an interest in the Data & Analytics space. So I’m continually being alerted to the presence of new providers attempting to add value in this way.
For example, in the last few week I’ve encountered users of and investors in various products which may have market-moving potential:
Clay - focused on improving lead generation (not just for financial services);
Inven - focused on improving company discovery;
Gain.pro - more accurate information on European companies.
Are they true Alpha? They might be. Like AltData, you have to suck it and see to know for sure. From the outside you can’t tell - every company claims to have the silver bullet.
You could argue though, that there’s a fourth (and perhaps blindingly obvious) way in which value is added to Company Data & Analytics: the quality and depth of the intelligence that is provided on each company.
And in this department, the best provider of Alpha on private companies are the Expert Networks.
As I said two newsletters ago:
Data & Analytics has a tendency to follow the money. Data providers produce datasets that companies want to buy. And the biggest spenders on data are financial services firms.
Another way of saying this is to repeat one of my favourite economic theories: former Google Chief Economist Hal Varian’s Rule:
A simple way to forecast the future is to look at what rich people have today; middle-income people will have something equivalent in 10 years, and poor people will have it in an additional decade.
In this extended metaphor:
Rich people = hedge funds
Middle-income people = private equity
Poor people = advisors, consultants and other professional services providers
Expert Networks began their existence serving the Hedge Fund industry but they have truly taken off in recent years assisting Private Equity firms to perform due diligence in their acquisitions of private companies.
Private Equity firms helped finance the growth of this sector beyond $2.1bn in revenues in 2022 - this figure includes the revenues of more than 120 expert networks.
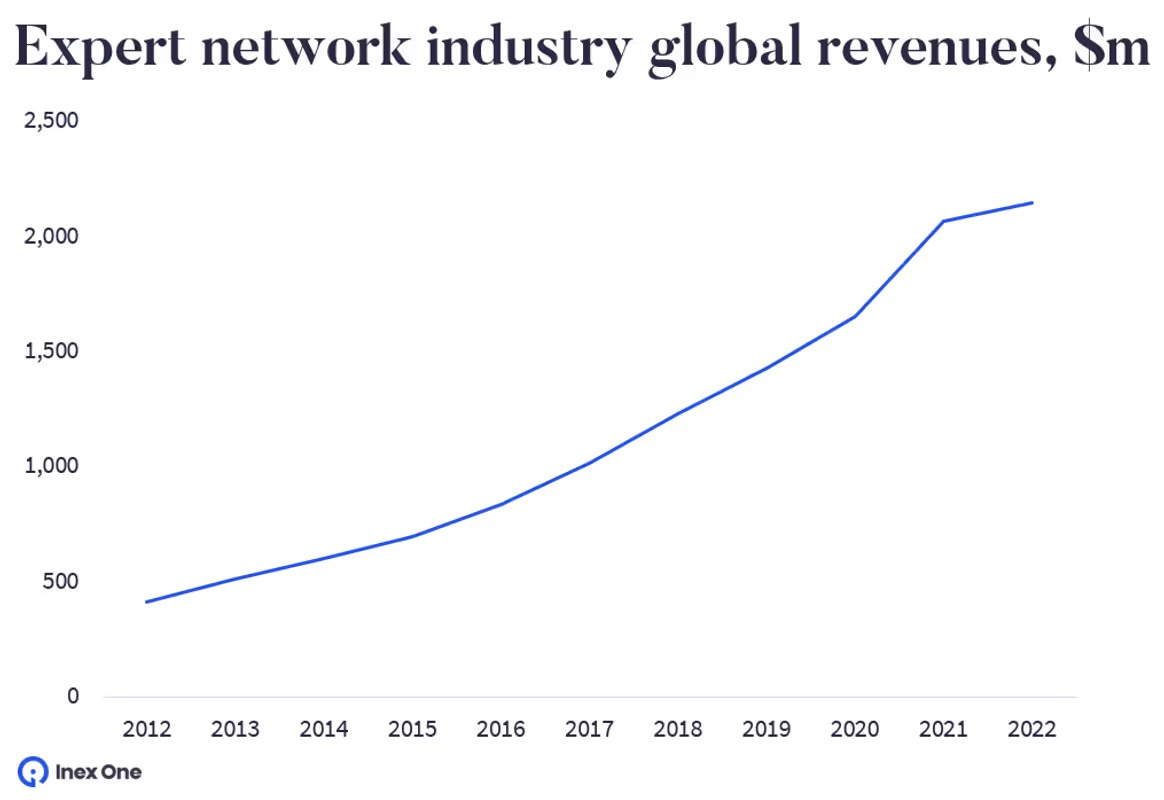
But Expert Networks have yet to truly penetrate the broader professional services community. Which suggests there’s further room to grow yet.
But this isn’t Data & Analytics, I hear you say.
True - the data produced by Expert Networks has traditionally been unstructured and has looked more like consultancy than subscription data. But this is changing.
Max Friberg, the CEO of Inex One, an Expert Network aggregator/consultancy, wrote an interesting article last year on the trend for Expert Networks to start to look like data businesses. He points out that ThirdBridge, one of the largest Expert Networks, has already repositioned itself as a data business. And that both Tegus and Alphasense, which I mentioned in my last newsletter as Matt Ober’s highlights for still-independent venture-scale AltData companies, are data-first expert networks. (At this point I need to get my kneepads out and apologise for dismissing them so quickly - they definitely are creating new data and I’m sure I’ll be talking about them again in a future post.)
So, in summary, many Expert Networks now provide subscription data products. I expect to see this trend develop as a) “middle-income” and “poor people” (to continue the analogy) demand access to their services and b) Expert Networks seek ways to serve the masses in a cost-effective way, while adding additional revenue streams to their businesses.
But, as we have seen, the data from these networks will also only remain Alpha data while not everyone has it. While it provides an edge. The slow descent to table stakes will then begin (if it has not already begun), and the hunt for new Alpha data will be under way (it surely already is).
Some things I’ve found interesting in the last week:
- Entrup in his It’s Pronounced Data newsletter. This one is a bit self-serving because he mentions this newsletter!
Interesting article in the New Yorker about how knowledge workers can navigate work in the information overload era.
Fascinating insight from the BBC into how Courtney Dauwelter deals with fear and pain in ultra-long distance endurance events.
Hi Alex,
Agree with Joe really loved the breakdown of discoverability, accuracy, and workflow. I work at Gain.pro and its a fascinating problem for us to solve especially discoverability and accuracy as we expanded our operations Globally. Looking forward to reading your work.
I love the breakdown of discoverability, accuracy, and workflow. I worked on this at CircleUp for a few years where we compiled data on private CPG companies. Fascinating problem to solve. Looking forward to reading more of your work